Quantum Leaps: Part 2
IARPA: Disbelief To Doubt Podcast
Episode 4: Quantum Leaps Part 2
Guest: Dr. Michael DiRosa
Transcript
[Music]
Intro: IARPA sponsors research that tackles the intelligence community's most difficult challenges and pushes the boundaries of science. We start with ideas that often seem impossible and work to transform them from a state of disbelief to a state of just enough healthy skepticism or doubt that by bringing together the best and brightest minds, we can redefine what's possible. This podcast will explore the history and accomplishments of IARPA through the lens of some of its most impactful programs and the thought leaders behind them. This is IARPA, Disbelief to Doubt.
Dimitrios Donavos: Welcome back to IARPA, Disbelief to Doubt. I'm your host, Dimitrios Donavos. In part two of this two-part episode, we continue our conversation with former program manager, Dr. Michael DiRosa. If you’re just joining us, make sure to go to iarpa.gov forward slash podcast to listen to part one of this episode where we cover some of the basics of quantum computing.
In part two, we discuss with Michael why quantum is such an IARPA hard problem, unpack IARPA’s history of quantum breakthroughs over the last decade, explore what applications might come from quantum computing in the future, and much more – including how Michael’s first foray into engineering and maybe even the world of quantum might of have had its roots in the mechanics of bowling alley pin setters. Take a listen.
Dimitrios Donavos: So, I think you've hinted at this as you've walked us through quantum computing, but I wanted to ask you in your own words, what makes quantum such an IARPA-hard problem?
Michael Di Rosa: This aspect for computing, for quantum information processing, it's a multidisciplinary endeavor. And if you want to make progress on it, in particular over this horizon, or at least a glimpse at it, of what does, to use a longer word or phrase, universal fault tolerant quantum computing look like, that figures in all the aspects of quantum error correction and conducting logic operations in a way that best preserves quantum coherence, keeps errors and faults from propagating. To do that, through that model, through that paradigm for quantum computing, draws on, well, you name it, physicists, material scientists, engineers, computer scientists, theoreticians’ mathematicians, all toward those kinds of objectives.
And they all need to be talking to one another because you can imagine that the theorist that comes up with the architectural paper needs to talk with the experimentalist to see if that's feasible. The experimentalists need to discuss what types of errors the real physical system has so that the theorists, who in this case don't mind getting their hands dirty working with experimentalists in real numbers, they might be able to arrange an architecture that is less vulnerable to the types of real noise that is present in these physical systems. So, it really is by that symbiosis that you can make progress in this particular field. And IARPA has a long history of having the types of programs where people from various backgrounds can join together on a team and make progress. And in that regard of...IARPA is a place right to make progress in a field like quantum computing.
Dimitrios Donavos: So, you've mentioned error corrected and fault tolerant quantum computing. Can you just step us through what exactly that means and why it is so important in terms of the focus of IARPA research in the quantum domain?
Michael Di Rosa: So, keeping in mind that this is the likely future for quantum computing, this mouthful of universal, full tolerant quantum computing. What that means is that the logic operations we normally think of as a programmer, I'm going to devise an algorithm and at some level of assembly language, I can distill it down to a handful of gates, not gates, controlled not gates and things like that. When I make those requests for logical gates through an algorithm. They'll be sent not to, they'll be acting on, I should say, more collectively, more holistically on the set of qubits encoded into a quantum error correction code called a logical qubit. And just that command of a gate will set in motion all these coordinated actions and choreography on the individual physical qubits that are composing the logical qubit, but to the programmer, what I understand to be my logical, my, my computation is taking place on these now abstract blocks called logical qubits. Underneath it, there's a lot of machinery sending commands to the physical qubits that compose a logical qubit, but to the program at some level, all I know is that I made a command on a logical qubit to carry out a gate either with it, you know, on, on itself or perhaps on a neighboring logical qubit.
Michael Di Rosa: So that's where the kind of quantum error correction comes in. Quantum error correction will keep, will preserve that quantum information within that block of a quantum error correction code. The aspect of fault tolerance comes in, well, because, you know, keeping logical qubits, you know, having them preserve logical information isn't enough. That's just memory. I need to compute, I need them to interact. So an easy way to do that possibly would be, if your time I want to gate with another logical qubit, I'm just going to unpack or unfold my logical qubit and have it interact in a simple way with the other logical qubit. And after that interaction, I'm going to fold them back up into their quantum error correction codes and proceed.
However, when you do that, you introduce the possibility of spreading errors, you know, too quickly, too quickly for your quantum error correction code to compensate. They can spread exponentially or geometrically, you know, one to two to four and so forth. So, the way that you can better preserve that quantum information and not build up errors to the failure of your quantum error correction code is interact logical qubits while keeping them in their quantum error correction codes. And that's not often easy. It does add a complication. It can be done. And, but that is also a necessary ingredient for maintaining, you know, this model for quantum computing. Have quantum error correction codes to clean up errors when they occur and also abide by say a policy or a rule of fault tolerance where I keep quantum information within those quantum error correction codes, don't unpack them so that they're preserved.
And there's other aspects of fault tolerance that are a little more nuanced. But for the most part, you have to conduct all the algorithms such that errors don't spread in some uncontrollable way. That's just the machinery of quantum computation that's to say nothing of course, you know, of the algorithms that are needed for gaining the computational efficiencies that quantum computation promises.
Dimitrios Donavos: The algorithms that are needed in order to derive these efficiencies are those things that are happening in the classical world that get applied into the quantum world? Or am I not thinking of that correctly?
Michael Di Rosa: This is a fascinating question. So, algorithms are what make computations effective. We use them all the time in our daily life with regular computers. So, quantum computers are not faster computers. I don't believe there's proof of some general superiority of quantum computers. To make quantum computers effective, you need to come up with clever algorithms that take advantage of this paradigm for computing. And again, in theory, and by the example I gave earlier, it can be effective. For say, physics problems or chemistry problems, to use those examples, computations there. that are intractable presently could be done far more effectively and quickly and such by instituting or finding, developing an algorithm that works with quantum computing. So that's the other hard part too, is learning how to work with quantum computing define effective algorithms and exactly why quantum computers provide in those particular instances the advantages they do.
That's also a topic of debate. Some might point to superposition, some might point to this uniquely, quintessentially quantum mechanical resource of entanglement, and there are other reasons. But nonetheless, just to boil it down to maybe a simple definition of effectiveness. If an algorithm is effective on a quantum computer, it will reduce the number of steps you would take to get some desired result to something substantially less than what it would take on a classical computer.
Dimitrios Donavos: And you mentioned error correction, which is important from the perspective of how IARPA has approached quantum computing and putting an emphasis on the need for error correction. And that leads us into the topic of just talking about IARPA in terms of being at the forefront of quantum research since 2009, when we launched the Coherent Superconductive Cubits program. Work funded by IARPA was named Science Magazine's Breakthrough of the Year in 2010. And I'm wondering if at a very high level, you can just walk us through some of the technological accomplishments that we've had in that area across our portfolio since that time?
Michael Di Rosa: Over that span from CSQ, to give it a shorter name, IA RPA has from the outset, has constructed programs that try to define, that always auger toward universal fault tolerant quantum computing. So, when CSQ started, well certainly when we bring up things like error correction codes, we know we're going to need more than one qubit. We're going to need, you know, several qubits, maybe a dozen qubits or more, and they all have to perform well. So, when CSQ was formed, there was already the recognition that yes, we need to make good qubits, but we need to make them better than what was available at the time and also make them in a way that lends itself to scalability and even reproducibility. So, the way the programs are built is yes, but yes, quantum computing, but do it this way.
Michael Di Rosa: Again, so it sort of begins to define and push that path for this universal fault tolerant quantum computing. I think the next program after that was MQCO or multi qubit coherent operations. So now we're going to throw in the C. Coherent. So great with CSQ, we've made some numbers of qubits. It happened to be for superconducting style of qubits. So yes, but now we're going to explore a variety of platforms. And after you make your platform of qubits, do something representative of a circuit with it, because we want to look for how the real world intervenes, invades, like through crosstalk and things like this. So do something in a mini algorithm that involves all the qubits you've meticulously fabricated or arranged.
So again, it takes another step, another, you know, keeps chipping away at this, at the obstacles, at this path for universal fault tolerant quantum computing. I should add that under CSQ, mean, a lot of this, it's this interplay of theory and technology as well. So, you also need technology and device physics to give this, give this inspection to, you know, to get up to the horizon and look beyond it. So, there were some technological devices that were quite enabling that were built under CSQ.
So, it begins to build kind of the community in the ecosystem and kind of like the overall technical apparatus, you know, on several technical platforms to proceed in this direction. Think there was another program called QCS quantum computing systems. And that was a look at, actually it was a lot of software development in QCS that asked the question of if you wanted to, let's say, you know, run a particular problem or an algorithm how well does your quantum processor have to perform? So, it's taking a look at, yes, you want to do something with your processor, but look at it from some fundamental perspective, modeling and software. What are your targets for the performance your devices have to be at? How many do you need? Things like that. So, I think that that set the stage for a more sort of computer science, you know, look at quantum computing. There was a lot of intensive work, you know, represented by CSQ and MQCO on developing the device physics.
Michael Di Rosa: But we also needed to know what metrics and benchmarks, you know, they would need to hit. And then after that came LogiQ, which said, great, technically we have the foundations now, enough qubits that we can think plausibly about running a very particular algorithm on them called quantum error correction. So, and that began in, I believe 2016. And at that time, I don't believe that there was error corrected logical qubit at the start.
And by the end of that program, roughly seven years later came for all the research teams, the four research teams, instances of error corrected logical qubits. So that was a prime instance of really putting theory into demonstrable practice. This worked. And I'd say over the string of programs, they continued to show that fundamentally as best we can peer down this path, you know, with the noise that we have and the apparatus we have, there's no real fundamental obstacle to quantum computing. Things are behaving again as best as we can inspect it, as we expect it to. So that all set the stage for now quantum information processing or the fundamental steps for that with quantum error correction codes or logical qubits.
Dimitrios Donavos: You've discussed our long history at IARPA in terms of quantum research, but I want to delve into what has motivated you to launch the ELQ program and what you hope to accomplish with that effort?
Michael Di Rosa: When I came to IARPA, it was to run the latter part of the program called LogiQ, which began with the objective of proving that these quantum error correction codes indeed could work and that fault tolerance also works. So, this was by a variety of technologies, putting theory, putting theory into practice. And by the same token, when theory and practice experimentalists get together, often something new arises. And I think by the end of logic, there was a much greater understanding of what quantum error correction means and not just how to demonstrate it in physical systems, but how to go about quantum error correction, perhaps more efficiently, how to study it, how to characterize it.
And so that was a success by the end of the program, but quantum information processing itself needs more. It needs logical qubits to interact and the complexity of that problem going from single logical qubits to two or more that interact to do something useful in the context of quantum information processing, you know, the next great leap, the next great objective for the field. So that was the inspiration behind starting ELQ or entangled logical qubits is to define a program that set that particular destination, set boundaries, set boundaries for that journey for maintaining fault tolerance and various other aspects that maintained relevance for the field of universal fault tolerant quantum computing, and by the program's end, show demonstrably an outcome for entangling logical qubits, again, this quintessential resource now done between logical qubits, not just physical qubits, and do so a way that we can report something we can measure, like the success in this case of using entanglement for teleportation, which turns out also to be necessary for quantum computation.
So overall, that was the goal for ELQ. Take the next great step beyond logic in using and developing logical qubits to the next sort of the next horizon for universal full-tolerant quantum computing. Entangling them, doing something demonstrably useful for quantum information processing for quantum computing, and do so with, by building an awareness for what the challenges beyond that will be. So, it's not an objective for itself. It's done in such a way that allows us, IARPA, the community in general, to know what the next challenges are ahead.
Dimitrios Donavos: What are we striving to achieve, thinking about the big picture with our quantum research at IARPA? And why is it important from a science perspective?
Michael Di Rosa: From an IARPA, and also from a science perspective, I think we're all trying to get our best view, our best sense of what this future is for computing. It's a model for computing and architecture that sits far apart from classical computing. We talked about some of the synergies about the care and feeding with classical computing, but in terms of how to handle quantum computing, how to think about it, how to, well, and also on the technology side, you we also need to learn what are the limitations, I would say, or of the technologies themselves. So, we need to know their limitations, what noise mechanisms they present, what noise mechanisms might be damaging, how they might be countered, in what ways with quantum error correction, how to predict the behavior of physical systems. So, there's a healthy interplay necessary when we try to glimpse this future of again, trying to implement a theory, put that into practice, but also knowing that the limitations of the technical expressions have to be fed back into the theory so that we could find a way that might either overcome these limitations or give experimentalist focus on what noise sources they need to combat.
Dimitrios Donavos: Tackling this very difficult IARPA hard problem requires this multidisciplinary, multi-team and worldwide effort that you've talked about. IARPA in its history has played a significant role in investing in quantum research and helping build out these communities that didn't necessarily exist. I want you to just think about what you think the legacy is going to be for IARPA's involvement in quantum?
Michael Di Rosa: That Legacy? Well, there's a longer story to this preceding IARPA, there was ARDA, the Advanced Research and Development Activity. And part of their charter, and this began in, I believe in the mid 1990s, late 1990s, and among their charter was to investigate the potential of quantum computing. So, they had programs in quantum computing. ARDA became the Disruptive Technology Office. And in 2007, when IARPA was stood up, DTO was absorbed into IARPA. So, in a sense, there was already a legacy of, of looking ahead to the future of quantum computing prior to IARPA. And that was continued through IARPA. So, to pick up with IARPA, they continued this particular pursuit, looking at universal fault tolerant quantum computing, building the technologies in cooperation with also the computer science and the theory necessary to work cooperatively with the experiments. So, I think the legacy for IARPA will be this this decided focus on the harder problem for the future for quantum computing. That the future needs to involve all the elements of theory and experiments now working side by side. And I think that has laid the foundation for the field and will continue to.
Dimitrios Donavos: Looking over the horizon, what kinds of applications from quantum computing do you hope to see in the next 10, 20 years?
Michael Di Rosa: One of the things I think quantum computing in the nearer term can do is aligned with those initial visionary ideas for quantum computing that would simulate physical systems and physics systems. It might be possible to answer, say, meaningful questions about physical systems through quantum computers. Does it behave in this way, which the outcome expected for this ensemble to get useful information and insights about physical systems that would be too tedious or laborious to do through experiment. So that, I think, is one of the things we could look forward to in the next decade or so, this aspect of simulating physical systems.
Dimitrios Donavos: And what are some of the applications that we might get from that simulation of systems? You talked a little bit earlier about chemistry and biology, and I'm curious if you could just expand on that so that our audience might understand what the potential is for quantum.
Michael Di Rosa: Depending on the, let's say for chemistry, often it's helpful to know what the, well this gets into some specifics about chemistry, but sometimes it helps for a complicated molecule to know exactly what its ground state energy is. It might help in areas of drug discovery with the folding behavior of a protein is. And to do so without, say, you know, all the types of trials that experiments would involve, that it might reduce the time it takes to come up with a particular solution that's workable, so that through quantum computing, you might be able to do some of the groundwork you would otherwise have to do with experiments.
Dimitrios Donavos: I want to put you in the position of being an adolescent reading a scientific novel that talks about this promise of quantum computing. And I want you to just imagine, if you were thinking of it from this perspective, this wide-eyed perspective, what would be a really impressive application of quantum sometime in the future that we might be talking about 40, 50 years from now?
Michael Di Rosa: At the very least, it'd be neat if this became maybe more than 50 years, but it became what we consider today to be just a regular calculator. Now, it doesn't mean you're just going to do simple arithmetic and everything else, but you know, let's say my homework problem is to go calculate a rather complicated response. It could be, again, in physics, it could be in any particular problem, it could be, I don't know, financial markets, whatever it might be.
And, it would become at that point, because we've been sort of accultured to think about things algorithmically, problems algorithmically, and how to take advantage of quantum information processing to solve that more efficiently, that I'd be able to set up the problem, and maybe that’s even, that comes rather intuitively, and get the answer that I would expect, or not that I would expect, but get the answer as simply as just pushing buttons on a calculator.
That would be fantastic. And leading up to that point again, is not just the technical underpinnings that makes a quantum computer possible wholesale shift in how we think about things algorithmically problems algorithmically and to take advantage of this so that we could approach this much like I'd say a top computer scientist approaches databases or, you know, particular problems today, not needing to know a lick of the physics that goes on within the machines that are doing the processing. But certainly, a very skilled approach in how to be resourceful at putting together the algorithms that accomplish particular objectives. So that's a tall order for 40 to 50 years from now, but I would hope that's where we're headed.
Dimitrios Donavos: So Michael, I want to give you the opportunity from a topic or scientific perspective to address any topics that we didn't cover today.
Michael Di Rosa: You've been quite thorough. I would just add the, perhaps the understatement that quantum computing is hard, but it's precisely that because not only is it hard, but it does seem as best we know to be sort of the, you know, the ultimate means for information processing. And it has some, it's too tantalizing to ignore. So as tantalizing as it is, it's also very hard. But I think that that's why it's been an extremely exciting arena for research.
Dimitrios Donavos: And fits right into IARPA's portfolio since hard is pretty much where we start think about funding IARPA programs. I want to close by just talking a little bit more about you personally, and I'd like you to just tell our audience something about you they might be surprised to learn?
Michael Di Rosa: Well, maybe, maybe it wouldn't be too surprising. Would you believe I was a bowling alley mechanic, employed bowling alley mechanic?
Dimitrios Donavos: Wow. No, I would not. Although with mechanical engineering in your sights, I guess it doesn't surprise me all that much. That sounds like a dangerous occupation.
[laughter]
Michael Di Rosa: It definitely was back behind the machines. Those could do some brutal things to you, any appendages, but it was a good learning ground as well for learning how things work and often why. Actually, one cool thing about that, it had its own form of information processing. It had really a mechanical computer in the back, and I was always fascinated to learn about that.
Dimitrios Donavos: I presume that when you were back there making any adjustments that you weren't under the threat of a bowling ball racing down the hardwood?
Michael Di Rosa: I wasn't going to give anybody an easy target, no.
Dimitrios Donavos: Okay.
[laugher]
Dimitrios Donavos: Describe a little bit what this mechanical computer looks like because now you've piqued my curiosity?
Michael Di Rosa: I'd say let's call it a gearbox, the size of a small automotive transmission. But back there were gears, levers, eccentrics, cams that would make the decision after you threw a ball. Of course, if you get a strike, what does it do? It sweeps away the pins and gives you a fresh set of 10. If you didn't get a strike, it would sweep away the pins and reset it, which you had left. And so that decision took place all mechanically. There were scissors that grabbed pins. If there were any, if they fully closed, if all of them closed, it made one decision. If one or more were open, it made another decision and spent a lot of time evaluating those, building them, rebuilding them. It was kind of fascinating what it could do. I mean, certainly machines afterwards were replaced by, by electronics, but there was a time, these were old pin setters, know, dating from the fifties when this was the most robust way to do it.
Dimitrios Donavos: Would it be a stretch to say that those were rudimentary gates that were opening and closing and that this was your first foray into quantum mechanics?
Michael Di Rosa: Indeed, it was.
Dimitrios Donavos: And Michael, what do you do for fun when you're not trying to solve some of the hardest problems in both the open scientific community and the IC?
Michael Di Rosa: Well, as immersed as I am in technology, I also enjoy being thoroughly unplugged. So, whether that's, you know, time in the West, you know, time away somewhere, usually where cell signals don't reach.
Dimitrios Donavos: I think all of us can appreciate that, especially in an era where we are overwhelmed with noise that comes from all of the interconnectivity that we have at our disposal. Michael, I want to thank you. This was a really enlightening and engaging conversation. I appreciate your time and insights today and look forward to hearing more as ELQ unfolds over the next several years.
Michael Di Rosa: Dimitrios, thank you. Absolute pleasure.
[Music]
Outro: Thank you for joining us. For more information about IARPA, and this podcast series, visit us at I-A-R-P-A.gov where you’ll find resources and citations for this and all of our episodes, including examples of quantum computing systems. You can also join the conversation by following us on LinkedIn and on X, formerly Twitter, at IARPA News.
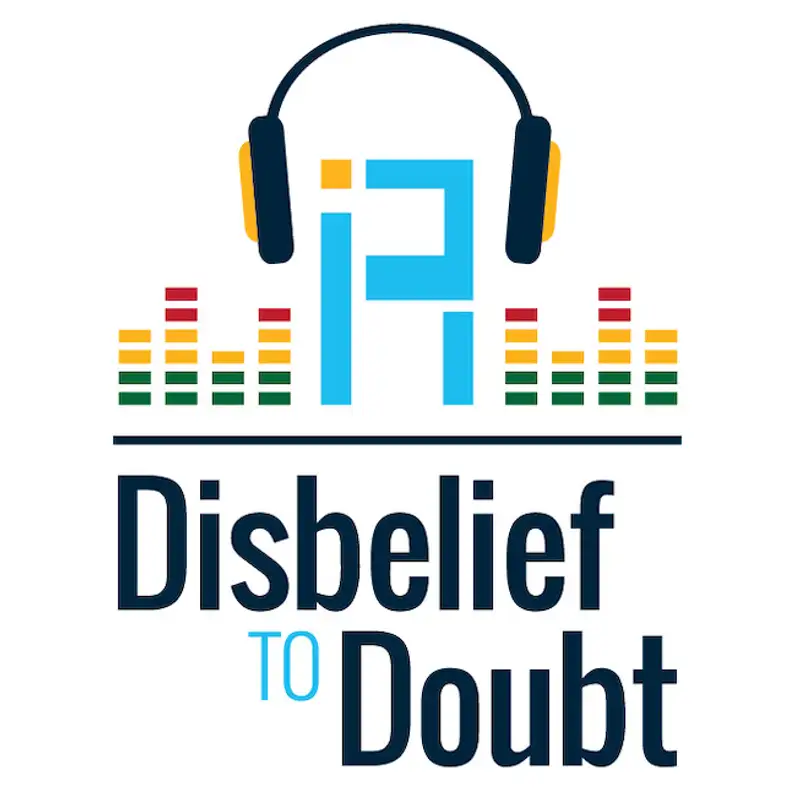