Quantum Leaps: Part 1
IARPA: Disbelief To Doubt Podcast
Episode 4: Quantum Leaps Part 1
Guest: Dr. Michael DiRosa
Transcript
[Music]
Intro: IARPA sponsors research that tackles the intelligence community's most difficult challenges and pushes the boundaries of science. We start with ideas that often seem impossible and work to transform them from a state of disbelief to a state of just enough healthy skepticism or doubt that by bringing together the best and brightest minds, we can redefine what's possible. This podcast will explore the history and accomplishments of IARPA through the lens of some of its most impactful programs and the thought leaders behind them. This is IARPA, Disbelief to Doubt. Welcome back to IARPA, Disbelief to Doubt.
Dimitrios Donavos Welcome back to IARPA Disbelief to Doubt. I’m your host, Dimitrios Donavos. In this episode, we explore the frontiers of physics by taking a journey through the quantum domain where a particle can be in two places at once and the rules of our everyday world don’t always apply. Quantum computing promises to revolutionize a host of industries by enabling rapid solutions to complex problems that are currently intractable for more traditional – or classical – computers.
As a primary funder of quantum research since 2009. IARPA sponsored efforts have resulted in multiple world-record demonstrations of quantum computing capabilities, including achieving a “Quantum Advantage” – when a quantum computer can solve a problem more efficiently than a classical one. IARPA funded quantum efforts have also led to over a thousand publications, dozens of patents, and the 2012 Nobel Prize in Physics.
In part one of this two-part episode, we sit down with former IARPA Program Manager, and resident Quantum expert, Dr. Michael DiRosa to talk about his journey to IARPA, what quantum computing is exactly, how it differs from more traditional computing, and much more. Take a listen.
Dimitrios Donavos: Dr. Michael DiRosa, welcome and thank you for joining us today on IARPA Disbelief to Doubt.
Michael Di Rosa: Thank you, Dimitrios. It's a pleasure.
Dimitrios Donavos: Michael, you have mechanical engineering degrees from Drexel, Penn State, and Stanford, and you arrived at IARPA via Los Alamos National Laboratory, where you were on assignment managing the Trapped Ion Quantum Computing Portfolio for the Laboratory for Physical Sciences, or LPS. Take us back to the beginning and walk us through what sparked your desire to pursue a career in mechanical engineering and how you ultimately ended up working on trapped ion quantum computing.
Michael Di Rosa: Perhaps like many from a young age, I was always interested in how things work. And so, I would take things apart, put them back together, usually all the pieces, and learn kind of experiential, you know, that way. And know, always kind of a mechanic about the house and also for some employment in my high school days, things like that, junior high. And so that kind of gave me the entry into mechanical engineering. It is...Gosh, as things went on, as you went over that arc going through from Drexel to Penn State to Stanford, increasingly, and always in the back of my mind, I was always interested in not only how things work, but why do they work? Why is this possible? And so, I think within that kind of came the transition to engineering and science, I think as perhaps I had always been, just one foot in each, the science from theory to math getting my hands dirty and trying to put it into practice. So, I've always tried to seek both, I suppose, you the practical side, but also kind of the far-reaching blue sky and what if that can be pulled in and put into practice.
Dimitrios Donavos: So, in discussing that arc, what ultimately led you to IARPA?
Michael Di Rosa: So, at Los Alamos, I was presented with the opportunity to join the Laboratory for Physical Sciences in their quantum computing group as a program manager running the Trapped Eye and Quantum Computing portfolio. And that was an interdisciplinary mix that I really enjoyed. Although as a program manager, it was an opportunity to set objectives in quantum computing for these particular physical systems. And that incorporated some of the engineering and physics I knew about for determined ends in quantum computing, at least for these physical systems. I returned to Los Alamos and then came the opportunity about a year and a half or two later to join IARPA as a program manager. And that was in truly IARPA fashion for a program. That was an opportunity to set an objective for the community in quantum computing. I undertook a program that was ongoing in quantum computing and as that was nearing its conclusion had an opportunity to extend that further into the next major leap.
But that really was a grand opportunity to set a destination for the community as a whole and define what that journey was going to be so it's more meaningful both to the community, to IARPA's missions and also have it meaningful as part of a national imperative for quantum computing. So that was a fantastic outlet.
Dimitrios Donavos: Along the way, who were some of your most influential people in your life, including your mentors, and how did they impact you professionally, personally?
Michael Di Rosa: Well, I guess over life, guess maybe I can count myself lucky as having perhaps too many to enumerate. And certainly, there were many through the educational sphere, through team sports and things like that. And they continued on into education and into my professional career. And I think there were those that certainly had every bit the enthusiasm and passion for their topic as they did the ability to treat it with humor and also humanity. And I think it's those that I recall most fondly and try to carry with me wherever I go.
Dimitrios Donavos: Where do you remember your first experience with quantum? And what about that motivated you to want to pursue that as a scientific topic of interest?
Michael Di Rosa: Again, it came kind of in that application of quantum mechanics and my graduate work increasingly began to draw on a field of laser spectroscopy, spectroscopy in general. And so that's often the science of learning about your environment through medium's response either to laser light or how the medium transmits that information in particular wavelengths of light. So, you can learn about what's there, what are the conditions, what reactions are going on and things like that. So to be able to combine or actually to learn about a medium through spectroscopy, you also need to know the basics of spectroscopy, sort of apply theory so that when you make a measurement, you can interpret that measurement into something that's meaningful. The temperature of a medium, the speed of a gas, the constituents that are in a reaction So increasingly I became really interested in learning as much as I could on both sides of that, the theory of spectroscopy and then also its applications.
Dimitrios Donavos: What excites you the most about being a program manager at IARPA?
Michael Di Rosa: I had spent time, let's say on the other side of the equation, the one actually writing proposals and doing the research. And with IARPA certainly came the opportunity to give back to that community, but in a different way, to create the opportunities that I had benefited from, both as a student and also in my professional career. And the other difference is that through being a program manager you get to give the field a nudge and a push in a particular direction. And more than that, you get to set a stage on which and give yourself a front row seat and also arrange what acts and how it's going to take place. And it's just also becomes a fascinating way also to learn through others. Certainly, in research you live vicariously, but it's a grand opportunity to set that opportunity for the field to go forward, to sort of unleash their creativity. So you're able to, with starting a program, being able to unleash all the resources and creative ideas and very good people in that particular field that you couldn't do by yourself, certainly.
Dimitrios Donavos: I think to that point, one of the advantages of being at IARPA is that the organization really brings together these communities across academia, government, industry, small business, in unprecedented ways to tackle these incredibly ambitious problems. And oftentimes, it's harnessing this technological talent across the globe towards solving a problem that has a significance for both the intelligence community and for the scientific community. What has working within those communities meant to you personally? And how do you see that effort continuing even after you leave IARPA and your tenure at the organization comes to a close?
Michael Di Rosa: It is important to, I mean, as you said, to harness really the best ideas internationally. If there's a particularly pressing problem and the science being democratized, it's good to tap into different approaches and not be so parochial about it. And one way to sort of enliven that range of approaches is to reach out internationally. So that's been really rewarding. As program manager, you can have the opportunity to take site visits to where the research is being done in this country and abroad and so that itself is quite educational. The different approaches that the different teams have, also the different way organizations run, that's all been really edifying for me, certainly. For the program itself, how that goes on and things at IARPA, I'd say that, you know, IARPA through the way it forms programs gives it structure for this type of model, you know, to continue to again, to reach out to, you know, solid groups, the best ideas wherever they might be to solve some of these problems. So, I'm confident of course that that would continue.
Dimitrios Donavos: When we look at the larger portfolio of research at IARPA, it's clear that the quantum programs have really resulted in the most robust publication history. And we've had significant achievements that have happened on the programs and high impact publications. For those folks that are listening, it's important to emphasize that when you work with IARPA, you maintain the ability to publish and that you have IP rights for your technology. How critical do you think that is in terms of the way performers are energized in terms of tackling such a difficult problem when they know that the fruits of their labor will actually be meaningful to the scientific community broadly?
Michael Di Rosa: Absolutely. On our programs, and I would think across IARPA, they should know that the work that they are doing, every bit of it could be put toward their own professional advancement. know, whatever inspires them in that way, whether it's publications, whether it's patents, even forming a startup company. All of that is their property. Every professional outlet is certainly available to them in the work they do for IARPA's programs.
Dimitrios Donavos: So, we talked a little bit here about accepting risk of failure. One of the things that we try and do at IARPA is when we do have technical failure, we try and learn from it and incorporate that into the next effort. I'm curious in your own career, what would you describe as one of your biggest failures and what did you learn about yourself from that experience?
Michael Di Rosa: I pause mostly because not that I haven't had failures. Certainly, there have been attempts to do particular things and they didn't quite pan out, whether in the laboratory, other things, but for me, that's always been a great learning opportunity. And figuring out, had, you perhaps, you embark on something and there's assumptions about why it ought to work and then it doesn't. And so, for me, that's often a path of inquiry into why it didn't work. And so, I don't see those failures that saves disappointments and perhaps that's not why, perhaps that's why they don't stick with me you know, indelibly, but there were always opportunities to learn. It's just part of the learning process.
Dimitrios Donavos: Well, when you're working with such a high-risk research, I think you have to be willing to accept that risk of failure and understand that you'll be learning from it and that learning will eventually inform an effort that hopefully will have some success as a consequence of that. I want to get into a little bit more on the theme of today's episode. And I want to start with very basic question. What exactly is quantum computing and how does it differ from conventional computing for our listeners who may not really fully appreciate the difference?
Michael Di Rosa: Sure. So, for quantum computing as a mode of computing, it is radically different than how we proceed with the Boolean algebra of classical computing. And let me just give an example, entirely theoretical, hypothetical at the moment. You know, one particular algorithm, you know, for quantum computing that is well known is for factoring large integers, factoring semi-primes. And it's the basis on which public cryptography is based. So let's take for granted some of the estimates that theorists are telling us under varying assumptions that to do this with a quantum computer would take, I'm going to introduce qubits, but would take about some tens of millions of qubits. And the algorithm itself would take some tens of billions of steps.
And after that, through this algorithm with a quantum computer, you would arrive at an answer to that particular problem. That if left to a classical computer would take, I don't know, by round estimate, a quadrillion years to solve. Yes!
Dimitrios Donavos: That's a long time.
Michael Di Rosa: That's a long time! You know, longer than the age of the universe. So that's what this is just in theory, what a quantum computer can do. But let me just give you an example of what that means for efficiency or exponential efficiency. So, we use two numbers. It was tens of millions of qubits. That sounds like a big number, but on our processors and our computer, you know, like a CPU, a central processing unit, has some billions of transistors, kind of the classical cousin of qubits. So, okay, you know, big numbers, but we're familiar with those types of big numbers. Well, it took tens of billions of steps. Well, we're actually familiar with those because those same processors on our laptops and such, they operated gigahertz rates. It's a, you know, a billion instructions a second.
So, this particular algorithm under those kinds of numbers that would solve what is a classically intractable problem into just seconds within a minute or so. Now there is no machine even remotely like that. It could certainly take, you know, decades, you know, to achieve.
So again, this is all on paper, but there is the allure then for quantum computing to be applied to other problems and perhaps on machines, you know, far less in scale to probably the earliest visionary ideas for using quantum computers. And that was for simulating physical systems, for simulating physics and related problems in chemistry, biology, medicine, say ranging from making inquiries into how could we arrive at superconductivity at room temperature? How could we discover drugs and cures faster than we do now? And perhaps some of those outlets bear fruit in the shorter term. So, there's this sense that quantum computing, well, I think it is not superior in every regard to classical computing, but there are some classes of problems where it might excel like that. And so that gives it impetus to discover what this frontier of computing might be like.
Dimitrios Donavos: So, the differences between quantum and classical computing are significant, but there's also this necessary symbiosis where you actually need both. Can you just describe to our audience what we mean by that?
Michael Di Rosa: A quantum computer, well, people tend to think of, there's quantum computing and it's going to be much like my laptop, or it's going to be like some supercomputer and it takes care of itself. But actually, as conceived presently, a quantum computer is going to take a lot of care and feeding from the classical world. The qubits themselves, they need control from the outside world. They need to be told what to do. So there's signals sending in controls for gates and such, all the logical operations that make the computation go. Qubits need to be measured. Often, depending on the type of algorithm used, they may have to be measured with some frequency. So, there's all this control from the outside world. And if we get into more complications, qubits are noisy. They don't fix themselves.
But there's a cure for that in the form of something called quantum error correction, where qubits are organized into ensembles that encode the states that you want to represent. And that also lend themselves to conducting or interacting, conducting gates, interacting with other logical qubits, carry out the logical gates that are represented in the computation or in the algorithm. That's all possible. Part of quantum error correction code
means that the qubit ensemble has to, at some frequency, at points in time, it has to present to the classical computer side a map, kind of a spatial temporal map of where it's, of what its measurements are reporting about errors. It's kind of an encoding of where the errors are. And so, on the classical compute side, it has to receive this map and it has to deduce from it exactly which qubits are in error and then go tell the control circuit to insert a gate or correction to go fix the errant qubit or one or more qubits. So, there's a lot going on to preserve what we could just loosely call the coherence of that system so that it lasts for the duration of the computation.
Dimitrios Donavos: So, I'm imagining that with a classical computer, I can go down to my local electronics store and buy one and bring it home and set it up on my desk. I doubt the way you're describing quantum computing you can do the same with a quantum computer. So, can you just describe a little bit about what a quantum computer looks like and what environment it typically runs in?
Michael Di Rosa: So, because this involves quantum mechanical states that are invested in physical devices preserving quantum behavior in all its coherence, you can imagine that these are special devices and they live in special environments. So, depending on the technology that's used, and again, qubits are physical things, just like transistors are physical things holding our binary logic, depending on the technology, they'll need to be kept at, put it mildly, very cold temperatures close to absolute zero. And then often they need to be kept, again, depending on the technology, in near perfect vacuum. So, these are not ordinary states. There's nothing you're going to put on your pocket or fold and take with you as you go walk around and have a conversation. So, they are very particular about the environment they need to be kept in, and that all is related to maintaining quantum coherence for as long as possible.
Dimitrios Donavos: So, one of the ways as a non-expert and somebody who's trained as a cognitive neuroscientist that I try and think about the difference between quantum more traditional computing is that quantum computers by their very nature handle uncertainty better than traditional computers do. That is to say that they are able to process information that traditional computers simply can't. And it sort of changes the scope of the problem for what a computer that is a quantum computer can tackle versus a traditional computer. What are some of the differences in terms of the types of problems that people can try and solve with a quantum computer?
Michael Di Rosa: You know, we're, we tend to think of, for these, it's often been said in these introductions is that, you know, a classical bit is zero or one and a quantum bit is both at the same time. Instead of thinking of it that way, again, maybe this is sort of just remove some of the gloss if that's possible of quantum computing. A qubit is every bit as precise, if a, as a classical bit, if a classical bit is being in either New York or Los Angeles, but nothing in between. A quantum bit could be in Omaha, Nebraska. Every bit is precisely as being in New York or Los Angeles. It's just that for us back in the human world, when we ask of it, finally, in some final disposition, we ask if it's either in New York or Los Angeles.
And then at that point, the quantum system will project itself into either. But before we ask that question of it, it's precisely where we put it. So, and this gets in the aspect of that quantum systems are delicate and they decohere and all this. So, they have their own difficulties of maintaining this precision. But again, the state of a, and it is a state for a quantum bit is every bit as precise as, again, theoretically, as what a classical bit would be, within its limitations of being zero or one.
So, the, you know, the types of algorithms you can perhaps do with quantum, they take advantage of, let's say this extra dimensionality that qubits present, that quantum algorithms present. And, you know, again, by the, you know, more conventional picture of how, or conventional history of how quantum computing developed, you know, was Feynman who and others who perhaps proposed that, if we want to calculate quantum mechanical systems, why don't we use quantum mechanical objects to do the computation? And so, they're quite efficient at describing the dimensional space necessary of a quantum system. And they can be put toward the gates and interactions necessary to kind of simulate quantum systems. So often they pose problems too large for a classical system to go simulate, but a quantum problem can be represented compactly within quantum objects and then they're put forward for quantum computation.
Dimitrios Donavos: So, when I think about quantum and I think about what makes quantum special, I think about the concepts of superposition and entanglement, which I think you've gotten a little bit into in your description there. In entanglement, if you flip two coins, they are independent of each other. The flipping in one has no bearing on the flipping of the other. But in entanglement, the qubits are linked and they influence each other. Can you unpack that a little bit for our audience and help us understand the significance of that and what that means in terms of what quantum computers can do as a function of that?
Michael Di Rosa: Yeah, for at least for quantum computation, what that really gets to is that there are these special correlations between two quantum objects. In this instance, the information in those two-quantum object is bound within those two objects. If you ask one of those two objects, which state it's in, like you said, if you just get a coin flip, you would just get, you know, just random information and if you were ignorant of that result and you go ask the other, you would again get, you know, an answer that was, you know, kind of random between two states, these so-called computational basis. But entanglement is making use of that special correlation between those two objects.
So, I wouldn't necessarily look at it as, you know, this spooky action at a distance, again, as it's sometimes cast as, but that there is an opportunity to take advantage of these special correlations called entanglement. So yeah, it is, I think, true that really some of the unique aspects in information processing and quantum computing does come by superposition and also entanglement. And the most successful algorithms will take advantage of those two.
Dimitrios Donavos: So, to frame it in a way that a non-expert might appreciate, I think about, you know, how a classical computer and a quantum computer might attempt a similar problem and have different sort of superpowers. So if we think about traversing a maze, a computer might traverse that maze, a traditional computer, by literally going through every single possible route through that maze, where a quantum computer could actually do it all at once in parallel. Is that an appropriate way to think about how a quantum and classical computer might tackle that problem differently?
Michael Di Rosa: Remember back to this, you know, when we talked about, you know, qubit having a precise value, anywhere between New York and LA. But when you ask it, you just get one answer. Am I in New York or in LA? After the algorithm runs and you ask a quantum computer an answer, it's just going to give you an answer. So, the, the analogy to think about for, let's say, solving this particular maze problem, as it were, the algorithm would have to be set up more like, you know, drawing on my you know, and what others do as well, drawing on like a spectroscopy analogy or an interferometer analogy. You construct your algorithm so that the right paths, if or maybe there's only one, they lead to some kind of constructive interference.
So, you get like a sharp peak in the answer when you finally read it out. The ones that dead end spiral into the middle, whatever, they destructively interfere. And so any answer, any peak that those types of answers or solutions kind of get suppressed. So that by the time you run this, and again, everything is in the algorithm, if everything runs well, the answer you get, the peak that you get will be at the solution you're after. So think of it in that way as a big interferometer, if that helps.
Dimitrios Donavos: That's a good analogy. So, if we think about superposition and entanglement as sort of the superpowers of quantum computers, what would be the kryptonite?
Michael Di Rosa: The world.
[Laugher]
Dimitrios Donavos:
You're gonna have to unpack that a little bit.
Michael Di Rosa:
The world. So yeah, I mean, you know, so it is, you know.
Dimitrios Donavos: The world is a noisy place.
Michael Di Rosa: It is a noisy place and so it's almost conspiratorial, mean there's like, there's this all this allure of computing, of quantum information processing through the fundamentals of quantum mechanics. yet, but these systems, they are also very sensitive to things of the outside world. And when I say the outside world, it is if you, you know, if you look at whatever is proposed as the quantum mechanical object that, you know, as the qubit, it's everything outside of it that is the world. So, you can't live in isolation. We need to control it. We need to ask it. We need to measure whatever the answer is. We need to set gates and everything else. So, we become the outside world. So almost invariably qubits are are going to decohere and so this is quite unlike again our classical bits where, I don't know, if you had a transistor and you, and I don't know, you had one of those cans of air and you gave it a puff of air on it or you waved it around or you carry your laptop on an airplane, it's perfectly fine.
I don't think you'll be doing that necessarily with, with a quantum computer. They have to be very well, very well protected. But then, but even then, because we intrude on what is potentially a perfect environment with to measure, to control, set gates and everything else. Then, you know, these, the systems are going to lose all those properties of coherence, superposition, you know, the coherence of entanglement and all that other stuff you know, quite quickly. so then, but there's hope. You know, there's hope.
There's actually, there's a few items in the list. mean, quantum mechanical objects, I know we kind of paint them as frail, fragile objects, but really when you look at like the energy, like the sort of the energy scales of, of, of some qubits, it's like, well, that's not that bad. I mean, you know, you know, for some for some qubits, you might need something the equivalent of, you know, like some bright light shining on it. Again, absent everything else, like, okay, that's not too bad. Unfortunately, it's also, you know, there's other forms of noise that might mess it up. It energetically doesn't look so bad. And then secondly, there is fortunately a form, know, forms of error correction that if errors, again, they, they, you know, invariably occur, but if they occur at a low enough rate and we can aggregate these qubits in particular ensembles that act like little machines to go detect errors, announce where the errors are, tell us where they are. And then we sort of, you know, puff in the correction. All this might hold together.
So, the, so the allure of quantum computing comes back, you know, quantum computing, you know, first, theoretically, it's like, great. These, there are some powerful algorithms, but it's never going to work. Qubits are fragile. But then it was revived with a lot of hope to say that's true, but there's quantum error correction and there's other aspects that go along with that. So all that's to say is that there is certainly, if not yet a technical path, certainly a theoretical path by which this can work.
[Music]
Outro: Thank you for joining us. For more information about IARPA and to listen to part two of this two-part series, visit us at I-A-R-P-A.gov. You can also join the conversation by following us on LinkedIn and on X, formerly Twitter, at IARPA News.
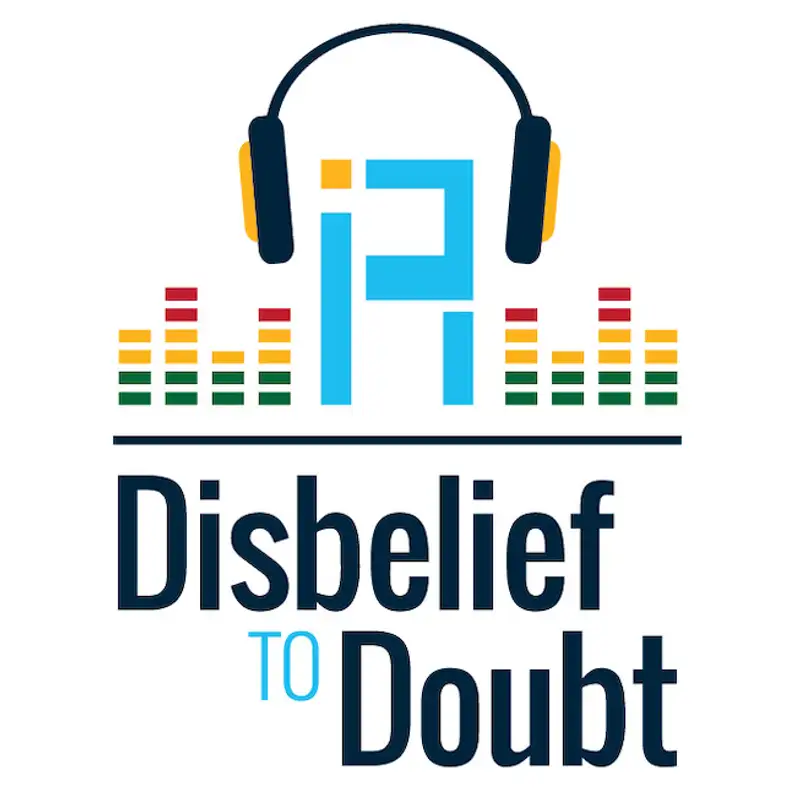